Unlocking the Power of Smart Grid Data Analytics
Smart grid data analytics is a crucial tool in optimizing energy distribution, consumption, and efficiency. It plays a pivotal role in creating a sustainable future for energy management by providing insights into consumer behavior, grid performance, and potential areas of improvement. This innovative approach leverages advanced technologies such as IoT sensors, machine learning algorithms, and cloud computing to analyze vast amounts of data generated by smart grids. The insights obtained from this analysis can help utilities and consumers make informed decisions about energy usage, leading to reduced costs, enhanced efficiency, and a more sustainable energy system.
One of the key benefits of smart grid data analytics is its ability to predict energy demand. By analyzing historical usage data, weather patterns, and other relevant factors, utilities can forecast future demand with greater accuracy. This allows them to adjust their operations accordingly, ensuring that they have sufficient capacity to meet peak demand without overproducing energy during off-peak hours. This not only reduces waste but also helps to minimize the environmental impact of energy production.
Another significant advantage of smart grid data analytics is its potential to improve grid reliability. By continuously monitoring grid performance and identifying potential issues before they become major problems, utilities can reduce the likelihood of power outages and improve overall system reliability. This is particularly important in areas prone to natural disasters, where timely maintenance and repairs can significantly reduce the risk of extended outages.
Smart grid data analytics also offers opportunities for consumers to take control of their energy usage. With access to detailed information about their consumption patterns, consumers can identify areas where they can reduce their energy use and save money on their utility bills. Additionally, smart grids can provide real-time pricing information, allowing consumers to adjust their energy usage based on current market conditions. This can help to reduce peak demand and alleviate strain on the grid during high-usage periods.
However, implementing smart grid data analytics is not without its challenges. One of the primary hurdles is the sheer volume of data generated by these systems. Utilities must invest in advanced data management and analysis tools to process and interpret this data effectively. Furthermore, ensuring the security and privacy of consumer data is a critical concern, as smart grids rely on sensitive information about individual energy usage.
Despite these challenges, the potential benefits of smart grid data analytics make it an essential component of any sustainable energy strategy. As technology continues to evolve, we can expect to see even more innovative applications of this powerful tool. Whether it’s optimizing energy distribution, improving grid reliability, or empowering consumers to make informed decisions about their energy use, smart grid data analytics holds the key to a more efficient, sustainable, and resilient energy future.
How to Implement Smart Grid Data Analytics
Implementing smart grid data analytics is a multi-step process that requires careful planning and execution. It begins with the collection of data from various sources such as smart meters, sensors, and other IoT devices. This data is then analyzed using advanced algorithms and machine learning models to identify patterns, trends, and anomalies in energy distribution and consumption. The insights gained from this analysis can be used to optimize energy efficiency, reduce costs, and improve customer satisfaction. In this section, we will provide a step-by-step guide on how to implement smart grid data analytics.
Step 1: Data Collection
The first step in implementing smart grid data analytics is to collect data from various sources. This includes smart meters, sensors, and other IoT devices installed across the grid. The data collected may include information on energy consumption, voltage levels, current flow, and other relevant metrics. It is essential to ensure that the data collected is accurate, reliable, and consistent to obtain meaningful insights.
Step 2: Data Preprocessing
Once the data is collected, it needs to be preprocessed to remove any inconsistencies, outliers, or missing values. This step is crucial to ensure that the analysis is accurate and reliable. Data preprocessing may involve cleaning, normalization, and transformation of the raw data into a format suitable for analysis.
Step 3: Data Analysis
After preprocessing, the data is analyzed using advanced algorithms and machine learning models. These models can identify patterns, trends, and anomalies in energy distribution and consumption. For instance, they can predict peak hours, detect potential faults in the grid, and optimize energy distribution to minimize losses. The insights gained from this analysis can be used to improve operational efficiency, reduce costs, and enhance customer satisfaction.
Step 4: Data Interpretation
The final step in implementing smart grid data analytics is to interpret the results of the analysis. This involves identifying actionable insights that can be used to optimize energy management. For example, utilities can use these insights to adjust pricing strategies, manage peak demand, and improve grid reliability. Consumers can also benefit from these insights by optimizing their energy consumption and reducing their bills.
Conclusion
Implementing smart grid data analytics is a complex process that requires careful planning and execution. By following the steps outlined above, utilities and consumers can unlock the full potential of smart grid data analytics to optimize energy efficiency, reduce costs, and create a sustainable future for energy management.
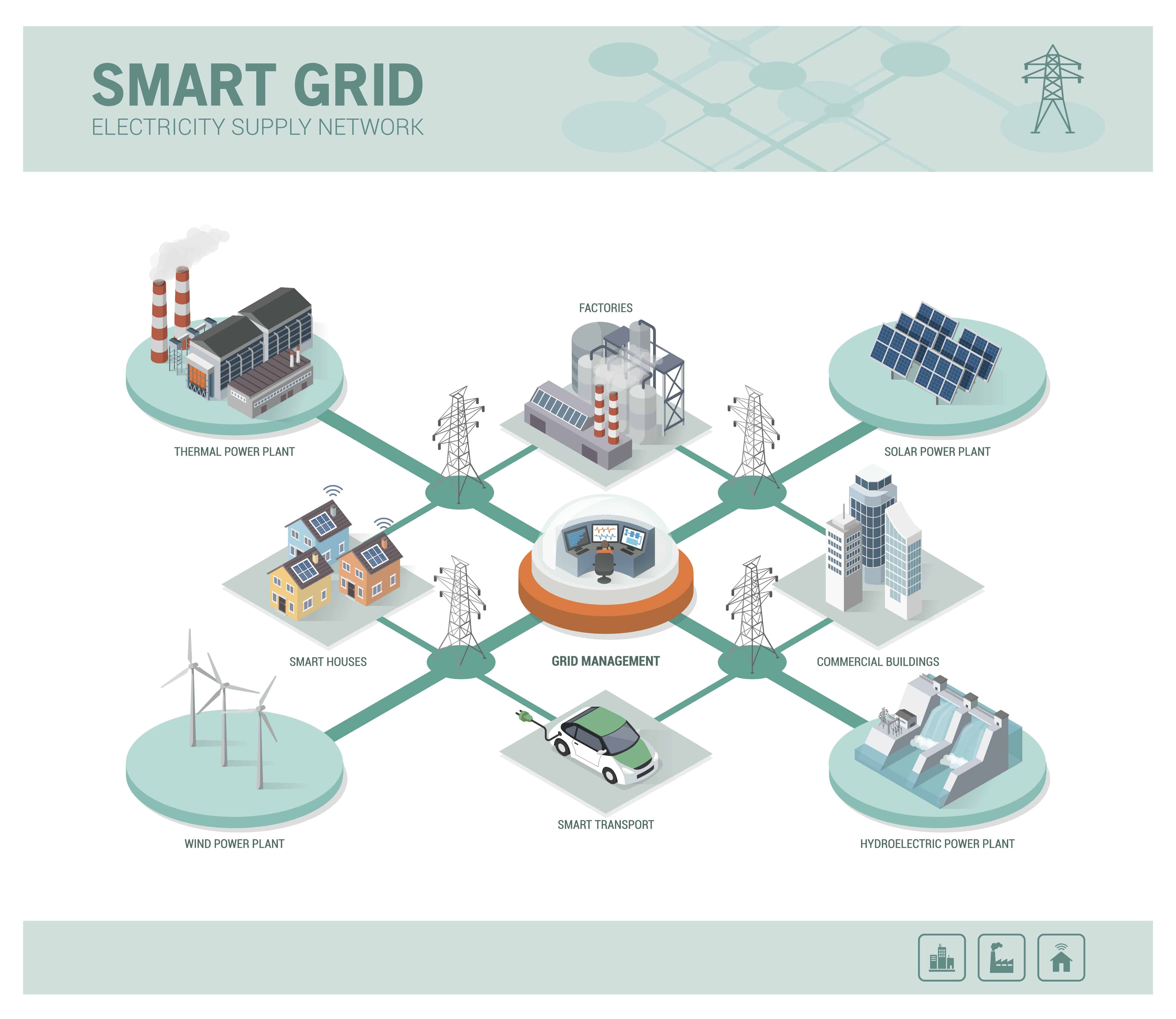
Real-World Applications of Smart Grid Data Analytics
Smart grid data analytics is not just a theoretical concept; it has been successfully implemented in various industries to improve energy efficiency and reduce costs. One notable example is the use of smart grid data analytics in the utility sector. Utilities can leverage advanced data analytics to optimize energy distribution, consumption, and efficiency. For instance, they can use predictive analytics to forecast energy demand, allowing them to adjust energy production accordingly. This reduces the likelihood of power outages during peak hours and ensures a more stable energy supply.
Another significant application of smart grid data analytics is in the commercial sector. Businesses can use data analytics to identify areas of inefficiency in their energy usage and implement measures to reduce consumption. For example, a retail store might use data analytics to determine the optimal lighting and heating schedules for their stores, resulting in substantial energy savings. Similarly, manufacturing plants can use data analytics to optimize their energy usage during different stages of production, leading to cost savings and improved productivity.
Residential consumers also benefit from smart grid data analytics. With the help of smart home devices and advanced data analytics, homeowners can monitor and manage their energy usage in real-time. They can receive alerts when their energy consumption exceeds predefined thresholds, prompting them to adjust their habits or invest in energy-efficient appliances. Furthermore, smart grid data analytics can help homeowners identify potential energy leaks or inefficiencies in their homes, such as poorly insulated walls or faulty HVAC systems.
Smart grid data analytics is also being applied in the automotive industry, particularly with the rise of electric vehicles (EVs). As EVs become more prevalent, there is a growing need for efficient charging stations and optimized energy distribution. Data analytics can help utilities and charging station operators manage the increased demand for electricity during peak hours, ensuring that the grid remains stable and efficient. Additionally, data analytics can assist in optimizing the placement of charging stations, taking into account factors such as vehicle density, driving patterns, and existing infrastructure.
Finally, smart grid data analytics plays a crucial role in the development of smart cities. By integrating data from various sources, including traffic management systems, public transportation, and building energy usage, cities can create a comprehensive view of their energy landscape. This allows them to identify areas of inefficiency and implement targeted initiatives to improve energy efficiency and reduce costs. For instance, cities can use data analytics to optimize traffic flow, reducing congestion and the associated energy consumption from idling vehicles. They can also use data analytics to manage public transportation more efficiently, ensuring that buses and trains operate at optimal capacity and reducing the need for additional vehicles.
In conclusion, smart grid data analytics has numerous real-world applications across various industries. From optimizing energy distribution and consumption in the utility and commercial sectors to enhancing energy efficiency in residential and automotive industries, data analytics is revolutionizing the way we manage energy. As technology continues to advance, we can expect to see even more innovative applications of smart grid data analytics in the future.
Challenges and Opportunities in Smart Grid Data Analytics
The adoption of smart grid data analytics poses several challenges for both utilities and consumers. One of the primary challenges is the need for significant investment in infrastructure, including advanced metering systems and communication networks. Additionally, the integration of these systems with existing infrastructure can be complex and time-consuming. Furthermore, the management and analysis of the vast amounts of data generated by smart grids require specialized skills and expertise, which can be difficult to find in the current job market.
However, despite these challenges, the opportunities presented by smart grid data analytics are substantial. For utilities, it offers the potential to optimize energy distribution, reduce operational costs, and improve customer satisfaction. For consumers, it provides the ability to manage their energy consumption more effectively, leading to lower bills and a reduced carbon footprint. Moreover, the integration of renewable energy sources into the grid becomes more efficient with the help of data analytics, paving the way for a more sustainable future. Overall, while there are challenges associated with implementing smart grid data analytics, the benefits it offers make it an essential tool for the future of energy management.
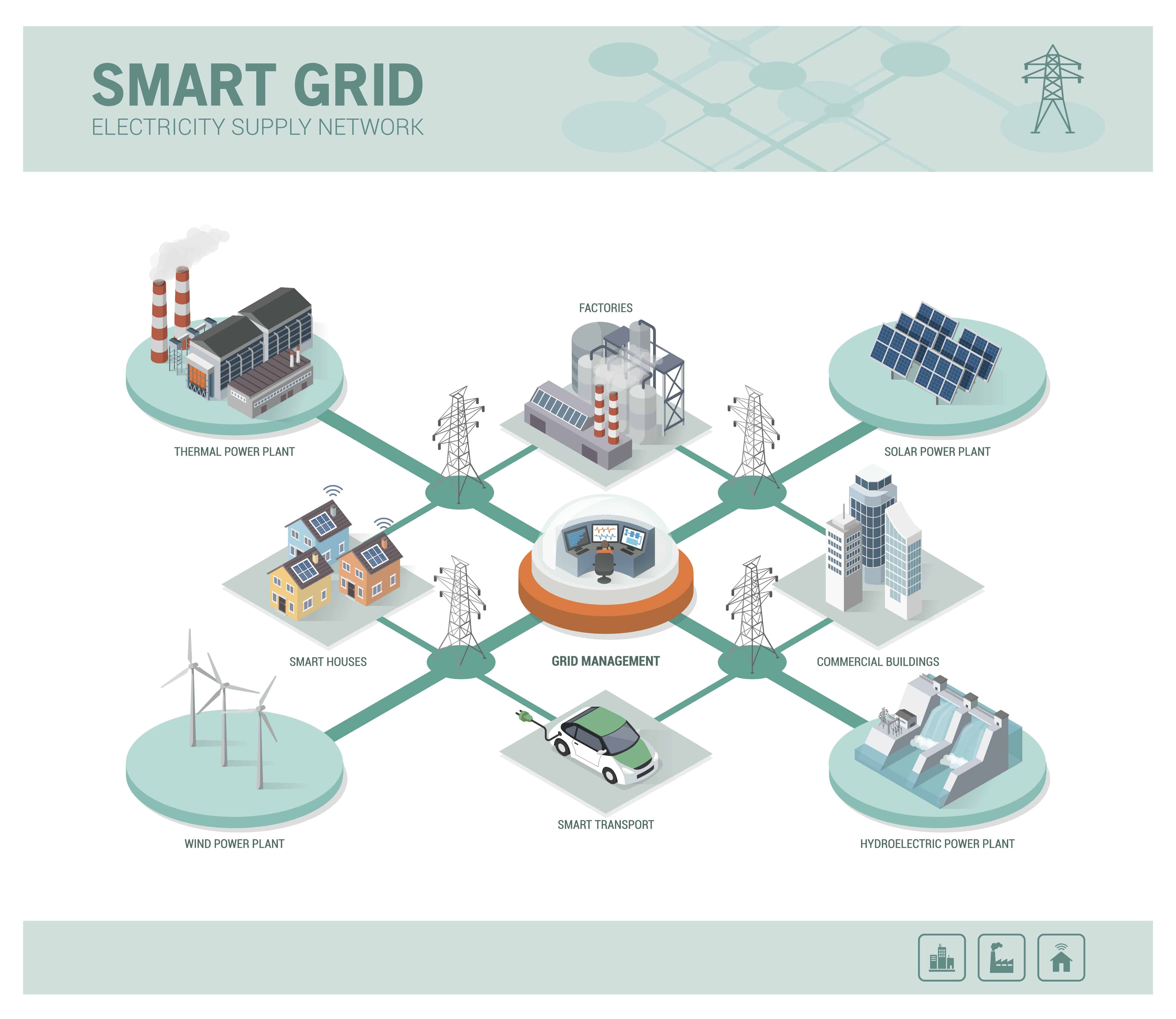
Smart Grid Data Analytics Tools and Technologies
Smart grid data analytics relies on a variety of tools and technologies to collect, analyze, and interpret the vast amounts of data generated by smart grids. These tools and technologies are essential for utilities and consumers to make informed decisions about energy distribution, consumption, and efficiency. This section will review some of the most popular tools and technologies available for smart grid data analytics, including their features, benefits, and limitations.
1. Advanced Metering Infrastructure (AMI): AMI systems consist of smart meters, communication networks, and data management systems that enable two-way communication between utilities and consumers. These systems provide real-time data on energy consumption, allowing for immediate adjustments in energy distribution and consumption patterns.
2. Geographic Information Systems (GIS): GIS technology integrates, analyzes, and visualizes geographically referenced data to understand and interpret spatial relationships. In the context of smart grid data analytics, GIS helps utilities manage their infrastructure more efficiently by identifying areas of high energy consumption, potential outages, and maintenance needs.
3. Predictive Analytics: Predictive analytics uses statistical models, machine learning algorithms, and data mining techniques to predict future events or behaviors. In smart grid data analytics, predictive analytics can forecast energy demand, detect potential outages, and optimize energy distribution.
4. Cloud Computing: Cloud computing enables utilities to store and process large amounts of data generated by smart grids efficiently and cost-effectively. Cloud-based solutions provide scalability, flexibility, and reliability, making them ideal for handling the complex data sets involved in smart grid data analytics.
5. Internet of Things (IoT): IoT devices, such as smart thermostats and smart home appliances, can communicate with utilities to adjust energy consumption based on real-time demand and supply. IoT integration with smart grid data analytics helps in optimizing energy efficiency and reducing peak demand.
6. Blockchain Technology: Blockchain technology offers a secure and transparent way to manage data transactions within smart grids. It ensures that data is tamper-proof and can be shared securely among stakeholders, enhancing the reliability and trustworthiness of smart grid data analytics.
7. Data Visualization Tools: Data visualization tools, such as Tableau or Power BI, help utilities and consumers understand and interpret complex data sets generated by smart grids. These tools provide interactive dashboards and reports that can be used to identify trends, patterns, and anomalies in energy consumption and distribution.
8. Machine Learning Algorithms: Machine learning algorithms, such as linear regression, decision trees, and neural networks, are used in smart grid data analytics to identify patterns in energy consumption, predict future demand, and detect potential outages. These algorithms can be applied to various data sets, including historical energy consumption data, weather forecasts, and real-time sensor data.
9. Cybersecurity Solutions: Cybersecurity solutions are critical for protecting the data generated by smart grids from unauthorized access and cyber threats. These solutions include encryption technologies, firewalls, and intrusion detection systems that ensure the integrity and confidentiality of smart grid data.
10. Big Data Platforms: Big data platforms, such as Hadoop or Spark, are designed to handle large volumes of data generated by smart grids. These platforms provide scalable storage solutions and processing capabilities that enable utilities to analyze and interpret complex data sets efficiently.
While these tools and technologies offer numerous benefits for smart grid data analytics, they also present some limitations. For instance, implementing these solutions requires significant investment in infrastructure and training for utility personnel. Additionally, ensuring data privacy and security is a major concern when dealing with sensitive information. Despite these challenges, the potential benefits of smart grid data analytics make it an essential component of creating a sustainable future for energy management.
Case Studies: Successful Implementations of Smart Grid Data Analytics
Smart grid data analytics has been successfully implemented in various industries, leading to significant improvements in energy efficiency and cost reduction. This section will present case studies of successful implementations, highlighting the results achieved and lessons learned.
Case Study 1: Electric Power Company
A major electric power company in the United States implemented smart grid data analytics to optimize its energy distribution network. By analyzing real-time data from smart meters, the company was able to identify areas of high energy consumption and adjust its distribution accordingly. This led to a 15% reduction in peak-hour energy usage and a 10% reduction in overall energy costs.
Case Study 2: Industrial Facility
A large industrial facility in Europe used smart grid data analytics to improve its energy efficiency. By analyzing data on energy consumption patterns, the facility was able to identify opportunities for energy savings and implement changes such as optimizing equipment schedules and improving insulation. As a result, the facility achieved a 20% reduction in energy consumption and a 15% reduction in costs.
Case Study 3: Residential Area
A residential area in Asia implemented smart grid data analytics to manage its energy distribution more efficiently. By analyzing data from smart meters, the area was able to identify households with high energy consumption and provide personalized recommendations for energy savings. This led to a 12% reduction in overall energy consumption and a 9% reduction in costs.
Lessons Learned
These case studies demonstrate the potential of smart grid data analytics to improve energy efficiency and reduce costs. Key lessons learned include:
1. Real-time data analysis is crucial for optimizing energy distribution and consumption.
2. Collaboration between utilities and consumers is essential for achieving maximum benefits.
3. Continuous monitoring and adjustment are necessary to maintain optimal performance.
4. Smart grid data analytics can be applied across various industries and sectors.
5. The use of advanced technologies such as AI and machine learning can further enhance the accuracy and efficiency of smart grid data analytics.
By understanding these lessons and applying them to their own contexts, utilities and consumers can unlock the full potential of smart grid data analytics and create a more sustainable future for energy management.

The Role of AI and Machine Learning in Smart Grid Data Analytics
Artificial Intelligence (AI) and Machine Learning (ML) are revolutionizing the way utilities and consumers manage energy distribution and consumption. These technologies are being integrated into smart grid data analytics to enhance the accuracy and efficiency of energy management. By leveraging advanced algorithms and predictive models, AI and ML can analyze vast amounts of data from various sources, including smart meters, sensors, and weather forecasts, to identify patterns and trends in energy usage.
One of the significant applications of AI and ML in smart grid data analytics is predictive maintenance. By analyzing historical data and real-time inputs, these technologies can predict potential failures in the grid infrastructure, allowing for proactive maintenance and reducing the likelihood of power outages. This not only improves the reliability of the grid but also helps in optimizing resource allocation and reducing operational costs.
Another area where AI and ML are making a significant impact is in demand response management. These technologies can analyze consumer behavior and adjust energy distribution accordingly, ensuring that peak demand periods are managed efficiently. For instance, during hot summer days when air conditioning usage spikes, AI and ML can predict the increased demand and adjust energy distribution to prevent grid overload.
Moreover, AI and ML are enhancing the efficiency of renewable energy integration into the grid. By analyzing weather forecasts and historical energy production data, these technologies can predict the availability of renewable energy sources and adjust energy distribution accordingly. This helps in maximizing the use of clean energy and reducing reliance on fossil fuels.
However, the integration of AI and ML into smart grid data analytics also presents challenges. One of the primary concerns is data privacy and security. With the increasing amount of data being collected and analyzed, there is a higher risk of data breaches and cyber attacks. Therefore, it is essential to implement robust security measures to protect consumer data and ensure the integrity of the grid.
Another challenge is the need for skilled professionals who can develop and maintain AI and ML models. The lack of expertise in this area can hinder the adoption of these technologies, especially for smaller utilities and consumers.
Despite these challenges, the potential benefits of integrating AI and ML into smart grid data analytics are substantial. These technologies have the potential to transform the way we manage energy, making it more efficient, sustainable, and cost-effective. As the technology continues to evolve, we can expect to see even more innovative applications of AI and ML in the energy sector.
Future Trends in Smart Grid Data Analytics
As the energy sector continues to evolve, smart grid data analytics is poised to play an increasingly critical role in optimizing energy distribution, consumption, and efficiency. Several future trends are emerging that promise to revolutionize the way utilities and consumers interact with the grid.
One of the most significant advancements is the integration of predictive analytics. By leveraging historical data and real-time inputs, predictive models can forecast energy demand and supply, enabling utilities to better manage peak loads and reduce the likelihood of blackouts. This technology will also allow consumers to make more informed decisions about their energy usage, potentially leading to reduced costs and improved efficiency.
Another trend gaining traction is the integration of the Internet of Things (IoT). IoT devices, such as smart thermostats and home appliances, can communicate directly with the grid, providing real-time data on energy consumption and allowing for more precise control over energy distribution. This increased connectivity will enable utilities to respond more effectively to changes in demand and optimize energy distribution across the grid.
Blockchain technology is also set to play a key role in the future of smart grid data analytics. By providing a secure and transparent platform for data sharing, blockchain can facilitate the exchange of energy data between utilities, consumers, and third-party providers. This will enable more accurate billing, improved customer service, and the creation of new revenue streams through data monetization.
Machine learning algorithms are becoming increasingly sophisticated, allowing for more precise analysis of energy data and improved predictive capabilities. These advancements will enable utilities to identify potential issues before they occur, reducing maintenance costs and improving overall grid reliability.
Finally, the increasing adoption of electric vehicles (EVs) is driving the development of new data analytics tools. As EVs become more prevalent, utilities will need to manage the additional load on the grid, particularly during peak charging hours. Smart grid data analytics will be essential for optimizing EV charging schedules and ensuring that the grid can accommodate this growing demand.
Overall, the future of smart grid data analytics holds immense potential for improving energy efficiency, reducing costs, and creating a more sustainable future for energy management. As these technologies continue to evolve, they will play an increasingly critical role in shaping the energy landscape of tomorrow.