Revolutionizing Renewable Energy with Intelligent Storage Solutions
The Future of Energy Storage: Leveraging Artificial Intelligence
As the world transitions to renewable energy, the importance of energy storage system optimization cannot be overstated. Traditional energy storage systems face significant challenges, including limited efficiency, high costs, and inadequate capacity. However, the integration of artificial intelligence (AI) is revolutionizing the energy storage landscape. Energy storage system optimization using AI has the potential to overcome these limitations and unlock a more sustainable future. By leveraging machine learning algorithms and data analytics, AI-driven solutions can enhance the performance, efficiency, and reliability of energy storage systems. This, in turn, can reduce greenhouse gas emissions, promote energy resilience, and support the widespread adoption of renewable energy sources.
Unlocking the Power of Data-Driven Insights in Energy Storage
The integration of artificial intelligence (AI) in energy storage systems has opened up new avenues for optimizing system performance. By leveraging the power of data-driven insights, AI can analyze vast amounts of data from energy storage systems, providing valuable insights into system performance, efficiency, and potential bottlenecks. This data analysis enables the identification of patterns and trends that would be difficult or impossible to discern through traditional methods. For instance, AI algorithms can pinpoint inefficiencies in charging and discharging cycles, allowing for adjustments that enhance overall system efficiency. Moreover, predictive analytics can forecast energy demand, enabling the optimization of energy storage and release. This not only improves the reliability of renewable energy sources but also reduces energy waste and enhances the lifespan of energy storage systems. As the global energy landscape continues to evolve, the role of AI in energy storage system optimization is set to become increasingly important, driving innovation and sustainability in the renewable energy sector.
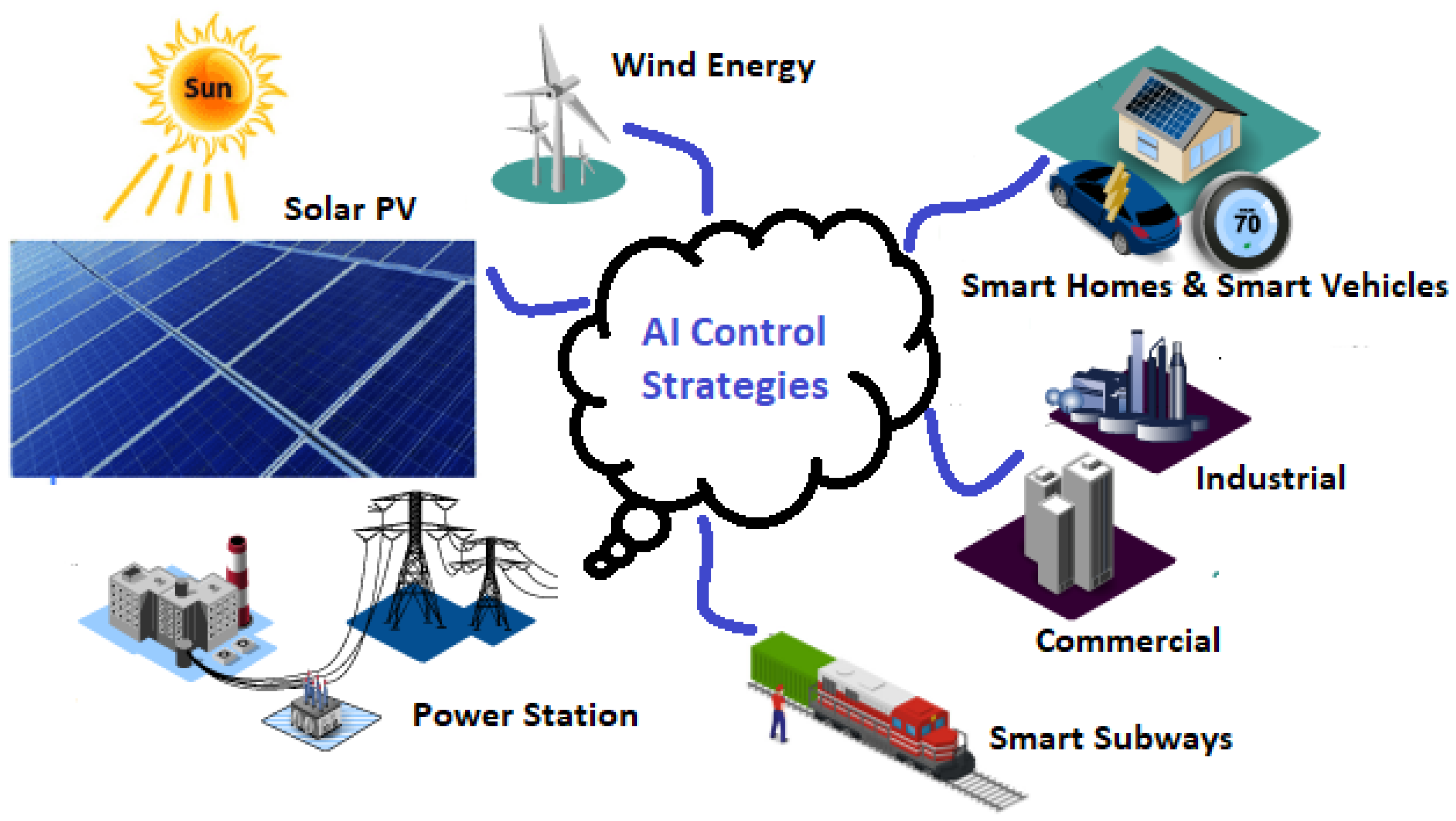
How to Optimize Energy Storage Systems with AI-Driven Forecasts
Energy storage system optimization using AI is revolutionizing the way we manage energy. By leveraging advanced algorithms and vast amounts of data, AI-driven forecasts can significantly enhance the efficiency and effectiveness of energy storage systems. This section will delve into the application of AI-driven forecasts in optimizing energy storage systems, providing insights into how AI can predict energy demand, optimize charging and discharging cycles, and reduce energy waste.
One of the primary challenges in energy storage system optimization is accurately predicting energy demand. Traditional methods often rely on historical data and basic statistical models, which can lead to inaccuracies and inefficiencies. AI-driven forecasts, on the other hand, utilize machine learning algorithms to analyze a wide range of data points, including weather patterns, time of day, and user behavior, to provide highly accurate predictions of energy demand. These forecasts enable energy storage systems to better prepare for peak demand periods, reducing the risk of energy shortages and improving overall system efficiency.
AI-driven forecasts also play a crucial role in optimizing charging and discharging cycles. By analyzing real-time data from energy storage systems, AI algorithms can identify optimal times for charging and discharging, ensuring that energy is stored and released efficiently. This not only extends the lifespan of energy storage devices but also maximizes their capacity, leading to improved system performance and reduced costs.
Another significant benefit of AI-driven forecasts in energy storage system optimization is the reduction of energy waste. Traditional energy storage systems often struggle to manage variable renewable energy sources, leading to energy being wasted during periods of low demand. AI-driven forecasts can help mitigate this issue by predicting periods of low demand and adjusting energy storage and release accordingly. This results in reduced energy waste and improved overall system efficiency.
Successful implementations of AI-driven forecasts in energy storage system optimization can be seen in various sectors. For instance, grid-scale energy storage systems are utilizing AI to predict peak demand periods and optimize energy storage and release. Similarly, electric vehicle charging infrastructure is leveraging AI to manage charging cycles and reduce strain on the grid during peak hours. Residential energy storage systems are also benefiting from AI-driven forecasts, enabling homeowners to optimize their energy usage and reduce costs.
In conclusion, AI-driven forecasts are a critical component of energy storage system optimization. By providing accurate predictions of energy demand, optimizing charging and discharging cycles, and reducing energy waste, AI can significantly enhance the efficiency and effectiveness of energy storage systems. As the global energy landscape continues to evolve, the role of AI in optimizing energy storage will become increasingly important, paving the way for a more sustainable and resilient energy future.
The Role of Deep Learning in Energy Storage System Optimization
Deep learning techniques have emerged as a promising tool in energy storage system optimization, offering unprecedented insights into complex data sets. These advanced algorithms, such as convolutional neural networks (CNNs) and recurrent neural networks (RNNs), are capable of analyzing large amounts of data from various sensors and devices within energy storage systems. This analysis can reveal intricate patterns and trends that may not be immediately apparent through traditional data analysis methods. By leveraging these insights, deep learning models can optimize energy storage system performance by identifying potential bottlenecks, predicting energy demand, and enhancing overall efficiency. For instance, CNNs can be used to analyze time-series data from energy storage devices, while RNNs can model sequential dependencies in energy usage patterns. The integration of deep learning techniques in energy storage system optimization has the potential to significantly reduce energy waste and improve the reliability of renewable energy sources.

Real-World Applications of AI-Optimized Energy Storage Systems
AI-optimized energy storage systems have numerous real-world applications that demonstrate their potential to revolutionize the way we manage and utilize energy. These applications span across various sectors, including grid-scale energy storage, electric vehicle charging infrastructure, and residential energy storage systems.
Grid-scale energy storage systems are critical for ensuring a stable and reliable grid, particularly as the integration of renewable energy sources continues to increase. AI-driven optimization can significantly enhance the efficiency and performance of these systems by analyzing historical data, weather forecasts, and real-time demand to predict energy needs and optimize charging and discharging cycles. This not only helps to balance the grid but also reduces the risk of power outages and improves overall system resilience.
Electric vehicle (EV) charging infrastructure is another area where AI-optimized energy storage systems can make a significant impact. As the adoption of EVs continues to grow, the strain on the electrical grid during peak charging hours has become a concern. AI-driven optimization can help mitigate this issue by analyzing driver behavior, vehicle usage patterns, and local energy demand to optimize EV charging schedules. This reduces the peak load on the grid, minimizes energy waste, and ensures that EV owners have access to reliable and efficient charging services.
Residential energy storage systems are also benefiting from AI-driven optimization. These systems typically consist of solar panels or wind turbines paired with a battery storage unit. AI algorithms can analyze weather forecasts, historical energy usage, and real-time energy demand to optimize the charging and discharging of the battery. This results in reduced energy bills for homeowners, improved system efficiency, and enhanced reliability during periods of high energy demand or grid outages.
Moreover, AI-optimized energy storage systems can play a crucial role in microgrids, which are small-scale, local energy systems that can operate in isolation from the main grid. These systems often rely on a combination of renewable energy sources and energy storage units. AI-driven optimization can help manage the distribution of energy within the microgrid, ensuring that each component operates efficiently and effectively to meet the needs of the community or facility it serves.
In conclusion, AI-optimized energy storage systems have the potential to transform various sectors by enhancing efficiency, reducing energy waste, and improving system reliability. As the technology continues to evolve, we can expect to see even more innovative applications of AI in the energy storage sector, ultimately paving the way for a more sustainable and resilient energy future.
Overcoming Challenges in AI-Driven Energy Storage System Optimization
While AI-driven energy storage system optimization holds immense potential, it is not without its challenges. One of the primary hurdles is data quality. The accuracy and reliability of AI models depend heavily on the quality of the data used to train them. In the context of energy storage systems, data quality issues can arise from various sources, including sensor malfunctions, data transmission errors, and inconsistencies in data formatting. To mitigate these challenges, it is essential to implement robust data validation and cleaning processes before feeding the data into AI models.
Another significant challenge is model interpretability. As AI models become increasingly complex, understanding how they make predictions becomes more difficult. This lack of transparency can hinder the adoption of AI-driven energy storage system optimization, as stakeholders may be hesitant to rely on models whose decision-making processes are not easily understood. To address this challenge, researchers and developers must focus on creating more interpretable AI models that can provide insights into their decision-making processes.
Cybersecurity is also a critical concern in AI-driven energy storage system optimization. As energy storage systems become more interconnected and dependent on AI, they become vulnerable to cyber threats. Hackers could potentially manipulate AI models to disrupt energy supply or steal sensitive data. Therefore, it is crucial to implement robust cybersecurity measures, such as encryption, secure communication protocols, and regular security audits, to protect AI-driven energy storage systems from potential threats.
Finally, the integration of AI-driven energy storage system optimization with existing infrastructure can be challenging. Many energy storage systems are legacy systems that were not designed with AI integration in mind. Upgrading these systems to accommodate AI-driven optimization may require significant investments in hardware and software. However, the long-term benefits of improved efficiency and reduced costs make these investments worthwhile.
Despite these challenges, the potential benefits of AI-driven energy storage system optimization make it an area worth exploring. By addressing these challenges through innovative solutions and continued research, we can unlock the full potential of AI in optimizing energy storage systems and accelerating the transition to renewable energy sources.

The Future of AI in Energy Storage System Optimization
As the global energy landscape continues to evolve, the role of artificial intelligence in optimizing energy storage systems is set to become increasingly pivotal. With the ongoing transition towards renewable energy sources, the need for efficient and reliable energy storage solutions has never been more pressing. In this context, AI-driven energy storage system optimization is poised to play a critical role in enhancing the efficiency, resilience, and sustainability of our energy systems.
In the future, we can expect significant advancements in AI technologies, including edge computing, transfer learning, and explainable AI, which will further enhance the capabilities of energy storage system optimization. Edge computing, for instance, will enable real-time data processing and analysis at the edge of the network, reducing latency and improving the responsiveness of energy storage systems. Transfer learning, on the other hand, will allow AI models trained on one energy storage system to be applied to others, accelerating the development and deployment of optimized solutions. Explainable AI, meanwhile, will provide insights into the decision-making processes of AI models, enhancing transparency and trust in AI-driven energy storage system optimization.
Moreover, the integration of AI in energy storage systems is expected to pave the way for autonomous energy storage systems. These systems will be capable of self-optimizing their performance based on real-time data inputs, ensuring maximum efficiency and minimizing energy waste. Autonomous energy storage systems will not only reduce operational costs but also enhance the overall reliability and resilience of the energy grid.
In conclusion, the future of AI in energy storage system optimization holds immense promise. As AI technologies continue to advance, we can expect to see even more innovative solutions emerge, driving the transition towards a more sustainable and efficient energy future.
As the world continues to transition towards renewable energy sources, the importance of efficient energy storage systems cannot be overstated. The integration of artificial intelligence (AI) in energy storage system optimization has emerged as a pivotal step in this journey, offering innovative solutions to enhance the efficiency, reliability, and sustainability of these systems. This article delves into the transformative potential of AI-driven energy storage system optimization, exploring its benefits, challenges, and future prospects.
Energy storage system optimization using AI is a multifaceted process that leverages advanced algorithms and machine learning techniques to analyze vast amounts of data from energy storage systems. By doing so, AI can identify patterns, predict energy demand, optimize charging and discharging cycles, and reduce energy waste. This not only improves the overall efficiency of energy storage systems but also enables them to adapt to changing conditions and user behaviors.
The application of AI in energy storage system optimization is particularly significant in the context of the global energy transition. As governments and industries worldwide strive to reduce greenhouse gas emissions and promote sustainable energy practices, the need for efficient energy storage solutions becomes increasingly urgent. AI-driven energy storage systems offer a promising avenue for achieving these goals, as they can optimize energy usage, minimize waste, and enhance the resilience of renewable energy grids.
Moreover, the integration of AI in energy storage system optimization can have far-reaching implications for various sectors, including electric vehicle charging infrastructure, residential energy storage, and grid-scale energy storage. By optimizing energy storage and consumption patterns, AI can help mitigate peak demand, reduce strain on the grid, and promote the widespread adoption of renewable energy sources.
However, despite the immense potential of AI-driven energy storage system optimization, several challenges must be addressed. Data quality issues, model interpretability, and cybersecurity concerns are just a few of the hurdles that researchers and practitioners face when implementing these systems. To overcome these challenges, it is essential to develop robust data management practices, improve model transparency, and enhance security measures.
Looking ahead, the future of AI in energy storage system optimization appears promising. Advances in edge computing, transfer learning, and explainable AI are expected to further enhance the capabilities of AI-driven energy storage systems, enabling them to become more autonomous, efficient, and adaptable. As these technologies continue to evolve, they will play an increasingly important role in shaping the future of renewable energy.
Ultimately, the significance of energy storage system optimization using AI lies in its potential to revolutionize the way we think about energy consumption and storage. By leveraging advanced algorithms and machine learning techniques, we can create more efficient, sustainable, and resilient energy systems that support the global transition to renewable energy. As we continue to explore the possibilities of AI-driven energy storage system optimization, it becomes clear that this technology holds the key to a cleaner, greener future for generations to come.