Understanding Big Analytics in the Cloud
Big analytics in the cloud ecosystem refers to the process of analyzing large and complex data sets using cloud-based platforms and tools. This approach has gained popularity due to its numerous advantages over traditional on-premises analytics solutions. Some of the key benefits include enhanced scalability, cost-effectiveness, and accessibility.
Scalability is a critical advantage of big analytics in the cloud. As data sets grow, cloud-based platforms can easily scale up resources to handle the increased workload. This flexibility is particularly beneficial for businesses that experience spikes in data generation or require rapid processing for time-sensitive insights.
Cost-effectiveness is another significant advantage of big analytics in the cloud. Organizations can avoid the upfront costs associated with purchasing and maintaining on-premises infrastructure by leveraging cloud-based services. Additionally, cloud providers often offer pay-as-you-go pricing models, allowing businesses to pay only for the resources they consume.
Accessibility is also improved with big analytics in the cloud. Users can access cloud-based platforms and tools from anywhere with an internet connection, enabling remote collaboration and real-time decision-making. This accessibility is particularly valuable for organizations with geographically dispersed teams or those requiring quick access to data-driven insights.
Throughout this article, we will explore various aspects of big analytics in the cloud ecosystem, including key players, best practices for platform selection, real-world applications, emerging trends, and challenges. By understanding these components, organizations can make informed decisions about adopting and implementing cloud-based big analytics solutions.

Key Players in the Cloud-Based Big Analytics Market
The cloud-based big analytics market is dominated by several major players, each offering unique solutions and services. These key players include Amazon Web Services (AWS), Microsoft Azure, Google Cloud Platform (GCP), and IBM Cloud.
Amazon Web Services (AWS) is a leading provider of cloud-based services, offering a wide range of big analytics tools such as Amazon Kinesis, Amazon Redshift, and Amazon SageMaker. AWS’s offerings cater to various analytics needs, from real-time data streaming to large-scale data warehousing and machine learning capabilities.
Microsoft Azure is another major player in the cloud-based big analytics market. Azure’s analytics services include Azure Databricks, Azure Synapse Analytics, and Azure Machine Learning. These tools enable users to process and analyze large data sets using familiar programming languages like Python, R, and SQL.
Google Cloud Platform (GCP) is known for its expertise in data analytics and machine learning. GCP offers tools such as BigQuery, Cloud Dataflow, and AutoML, which enable users to analyze massive data sets and build machine learning models with minimal coding expertise. GCP’s user-friendly interface and seamless integration with other Google services make it an attractive option for many organizations.
IBM Cloud is a comprehensive cloud platform that offers various big analytics tools, including IBM Watson Studio, IBM Cloud Pak for Data, and IBM Db2 Warehouse. These tools cater to various analytics needs, from data science and machine learning to data warehousing and business intelligence.
When selecting a cloud-based big analytics platform, organizations should consider factors such as data security, pricing models, ease of use, and integration capabilities. For instance, AWS offers flexible pricing models, while Azure provides seamless integration with Microsoft Office products. GCP is renowned for its user-friendly interface, and IBM Cloud offers robust security features. By carefully evaluating these factors, organizations can choose the most suitable cloud-based big analytics platform for their unique needs.

How to Choose the Right Cloud-Based Big Analytics Platform
Selecting the most suitable cloud-based big analytics platform is crucial for organizations seeking to harness the power of big data. To make an informed decision, consider several factors, including data security, pricing models, ease of use, and integration capabilities. Here, we discuss each factor in detail and mention specific tools or features offered by different providers.
Data Security
Data security is paramount when selecting a cloud-based big analytics platform. Providers like Amazon Web Services (AWS), Microsoft Azure, Google Cloud Platform (GCP), and IBM Cloud offer robust security features, including encryption, access controls, and compliance with various industry standards. Ensure that the chosen platform adheres to your organization’s security policies and regulatory requirements.
Pricing Models
Pricing models for cloud-based big analytics platforms vary. Some providers, like AWS, offer pay-as-you-go pricing, while others, like Microsoft Azure, provide subscription-based models. Consider your organization’s budget and analytics needs when selecting a pricing model. Additionally, evaluate any hidden costs, such as data transfer fees or storage expenses, to avoid unexpected charges.
Ease of Use
Ease of use is another critical factor when choosing a cloud-based big analytics platform. Providers like Google Cloud Platform (GCP) and IBM Cloud offer user-friendly interfaces, making it easier for users with limited technical expertise to navigate and analyze data. On the other hand, platforms like Amazon Web Services (AWS) and Microsoft Azure cater to both beginners and experienced users, providing advanced tools and features for data analysis.
Integration Capabilities
Integration capabilities are essential when selecting a cloud-based big analytics platform. Ensure that the chosen platform integrates seamlessly with your existing systems and tools. For instance, Microsoft Azure integrates well with other Microsoft products, while Google Cloud Platform (GCP) offers seamless integration with other Google services. Similarly, AWS and IBM Cloud provide extensive integration capabilities with various third-party tools and services.
By carefully evaluating these factors, organizations can choose the most suitable cloud-based big analytics platform for their unique needs. Remember that the right platform should offer robust security features, flexible pricing models, user-friendly interfaces, and seamless integration capabilities to ensure successful big data analytics.
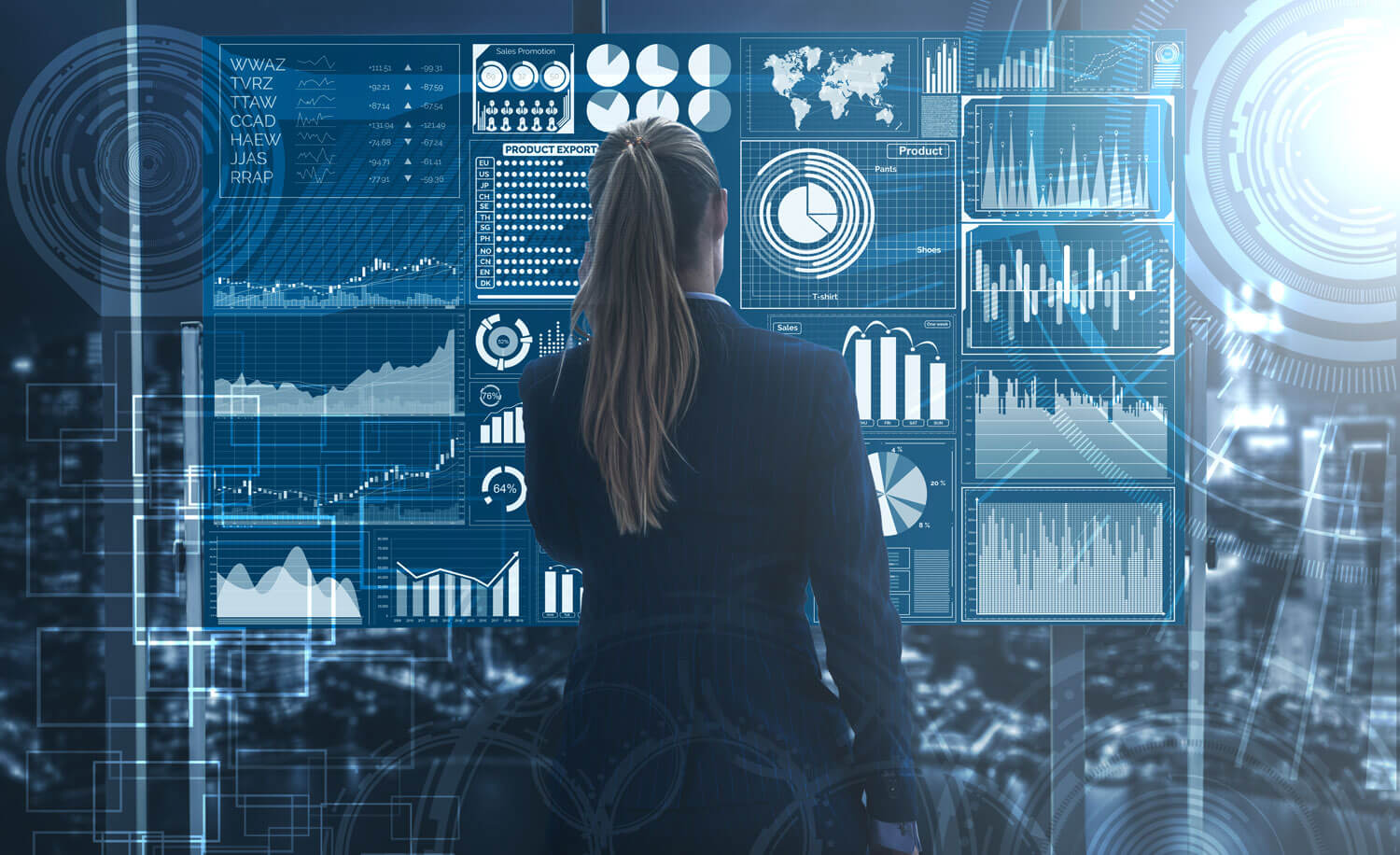
Real-World Applications of Big Analytics in the Cloud
Big analytics in the cloud ecosystem has revolutionized various industries by enabling organizations to harness the power of big data effectively. This section highlights some real-world applications of big analytics in the cloud, such as predictive maintenance, fraud detection, and customer segmentation.
Predictive Maintenance
Predictive maintenance is a proactive approach to maintaining equipment and systems by analyzing historical data and identifying patterns that indicate potential failures. Big analytics in the cloud enables organizations to collect, store, and analyze vast amounts of data from various sources, including sensors, machines, and enterprise systems. For instance, a manufacturing company can use big analytics in the cloud to monitor equipment performance, predict failures, and schedule maintenance, thereby reducing downtime and maintenance costs.
Fraud Detection
Fraud detection is another area where big analytics in the cloud has made significant strides. By analyzing large datasets, organizations can identify patterns and anomalies that indicate fraudulent activities. For instance, financial institutions can use big analytics in the cloud to monitor transactions, detect unusual patterns, and prevent fraud. Big analytics in the cloud enables real-time fraud detection, which is critical in industries where fraud can have severe consequences.
Customer Segmentation
Customer segmentation is the process of dividing customers into groups based on common characteristics, such as demographics, behavior, and preferences. Big analytics in the cloud enables organizations to collect and analyze vast amounts of customer data from various sources, including social media, web analytics, and enterprise systems. By analyzing this data, organizations can gain insights into customer behavior, preferences, and needs, enabling them to tailor their marketing strategies, improve customer engagement, and increase customer loyalty.
These are just a few examples of how big analytics in the cloud has transformed various industries. By enabling organizations to harness the power of big data, big analytics in the cloud has created new opportunities for innovation, growth, and competitiveness. As the cloud ecosystem continues to evolve, we can expect to see even more exciting applications of big analytics in the cloud.
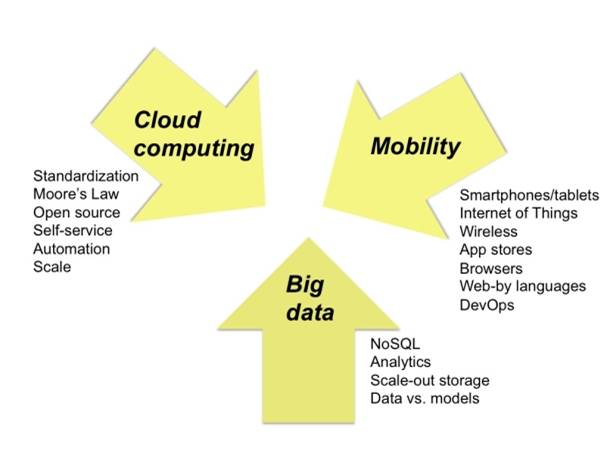
Emerging Trends in Cloud-Based Big Analytics
The cloud-based big analytics market is constantly evolving, with new trends and technologies emerging regularly. This section discusses some of the most significant emerging trends in cloud-based big analytics, such as serverless computing, artificial intelligence (AI), and machine learning (ML).
Serverless Computing
Serverless computing is an emerging trend in cloud-based big analytics that enables organizations to build and run applications without worrying about infrastructure management. Serverless computing allows developers to focus on writing code and creating features, while the cloud provider manages the underlying infrastructure. This approach can lead to increased efficiency, scalability, and cost-effectiveness, making it an attractive option for big analytics in the cloud ecosystem.
Artificial Intelligence (AI) and Machine Learning (ML)
AI and ML are transforming the big analytics landscape in the cloud ecosystem. By enabling machines to learn from data, AI and ML can help organizations make more informed decisions, automate processes, and gain a competitive edge. Big analytics in the cloud ecosystem can benefit from AI and ML in various ways, such as predictive analytics, natural language processing, and computer vision. For instance, a retail company can use AI and ML to analyze customer data, predict buying behavior, and personalize marketing campaigns.
These are just a few examples of how emerging trends in cloud-based big analytics are shaping the future of the industry. As the cloud ecosystem continues to evolve, we can expect to see even more innovative and exciting applications of big analytics in the cloud. Organizations that stay up-to-date with these trends and invest in the right technologies can gain a competitive edge and drive growth and innovation.
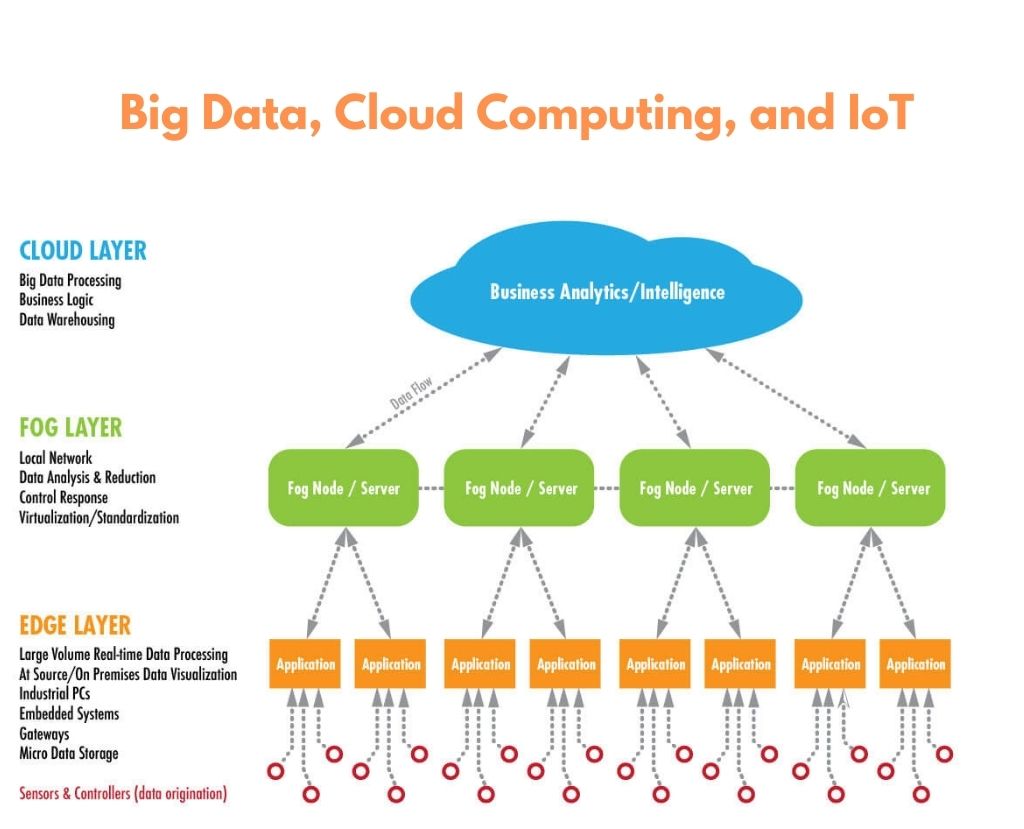
Challenges and Best Practices in Implementing Big Analytics in the Cloud
Implementing big analytics in the cloud ecosystem can be a complex process, with several challenges that organizations need to overcome. Here are some common challenges and best practices to help organizations successfully implement big analytics in the cloud.
Data Privacy
Data privacy is a significant concern for organizations implementing big analytics in the cloud. To address this challenge, organizations should ensure that they comply with data protection regulations and standards. They should also implement robust data encryption, access controls, and monitoring to prevent unauthorized access and data breaches.
Migration Complexities
Migrating big analytics workloads to the cloud can be complex, especially for organizations with legacy systems and data architectures. To overcome this challenge, organizations should conduct a thorough assessment of their existing data infrastructure and applications to identify potential migration issues. They should also work with experienced cloud service providers and migration experts to ensure a smooth and successful migration.
Talent Acquisition
Implementing big analytics in the cloud requires specialized skills and expertise. Organizations may face challenges in acquiring and retaining talent with the necessary skills to implement and manage big analytics in the cloud. To address this challenge, organizations should invest in training and development programs to upskill their existing workforce. They should also consider partnering with cloud service providers that offer managed services and support.
Best Practices
Here are some best practices for implementing big analytics in the cloud:
- Develop a clear and comprehensive cloud adoption strategy that aligns with your business objectives and data analytics needs.
- Choose a cloud service provider that offers robust security, scalability, and flexibility features.
- Implement a data governance framework that ensures data quality, accuracy, and consistency.
- Monitor and optimize your cloud usage and costs regularly to avoid overspending.
- Invest in training and development programs to upskill your workforce and build a data-driven culture.
By following these best practices, organizations can successfully implement big analytics in the cloud and leverage its benefits to drive growth, innovation, and competitiveness.
The Future of Big Analytics in the Cloud Ecosystem
The cloud ecosystem has revolutionized the way businesses operate, and big analytics is no exception. As we look to the future, advancements in technology and changing business needs will continue to shape the big analytics landscape in the cloud ecosystem.
One of the most significant trends in cloud-based big analytics is the increasing adoption of artificial intelligence (AI) and machine learning (ML) technologies. AI and ML algorithms can analyze vast amounts of data quickly and accurately, enabling businesses to make more informed decisions and predictions. By leveraging these technologies, organizations can gain a competitive edge and drive innovation.
Another trend that is shaping the future of big analytics in the cloud ecosystem is the move towards serverless computing. Serverless computing enables businesses to build and run applications without having to manage servers or infrastructure. This approach can help organizations reduce costs, increase scalability, and improve agility. By adopting serverless computing, organizations can focus on developing applications and delivering value to their customers.
In addition to these trends, the big analytics market in the cloud ecosystem is expected to grow significantly in the coming years. According to a recent market research report, the global cloud-based big data analytics market is expected to reach USD 65.4 billion by 2027, growing at a CAGR of 21.7% during the forecast period. This growth is being driven by the increasing adoption of cloud-based big analytics solutions by businesses of all sizes and industries.
As the big analytics market in the cloud ecosystem continues to evolve, organizations must be prepared to adapt to changing technologies and business needs. To stay competitive, organizations should invest in training and development programs to upskill their workforce and build a data-driven culture. They should also develop a clear and comprehensive cloud adoption strategy that aligns with their business objectives and data analytics needs.
In conclusion, the future of big analytics in the cloud ecosystem is bright, with advancements in technology and changing business needs driving growth and innovation. By investing in training, building a data-driven culture, and developing a clear cloud adoption strategy, organizations can leverage the power of big analytics in the cloud ecosystem to drive growth, innovation, and competitiveness.
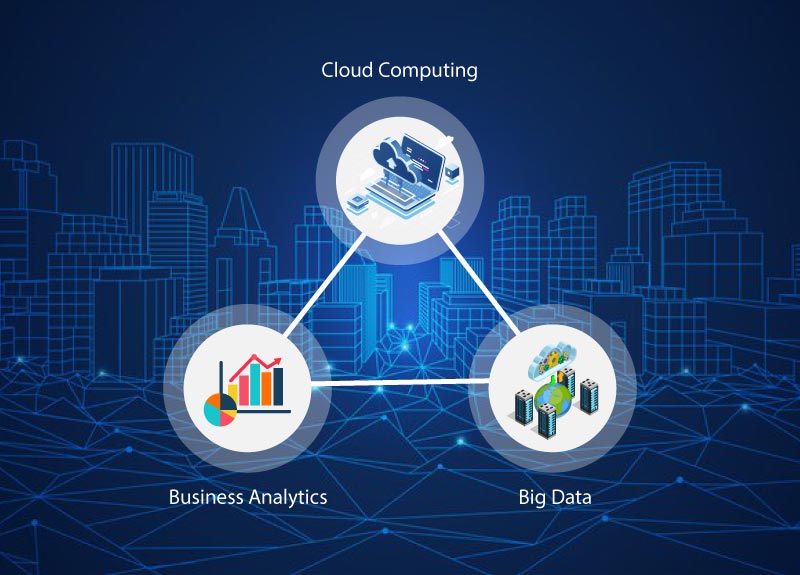
Preparing Your Organization for the Adoption of Big Analytics in the Cloud Ecosystem
As big analytics in the cloud ecosystem becomes increasingly popular, many organizations are looking to adopt these solutions to gain a competitive edge. However, adopting big analytics in the cloud ecosystem requires careful planning and preparation. In this article, we will provide guidance on preparing an organization for the adoption of cloud-based big analytics.
First and foremost, it is essential to have a clear strategy for adopting big analytics in the cloud ecosystem. This strategy should align with the organization’s overall business objectives and data analytics needs. It should also consider the potential challenges and risks associated with adopting cloud-based big analytics solutions.
Once the strategy is in place, it is crucial to invest in training and development programs to upskill the workforce and build a data-driven culture. This can include training on data analytics tools and techniques, as well as training on the specific cloud-based big analytics platform that the organization has chosen.
When selecting a cloud-based big analytics platform, it is essential to consider factors such as data security, pricing models, ease of use, and integration capabilities. It is also important to consider the specific tools and features offered by different providers. For example, some providers may offer advanced AI and ML capabilities, while others may offer more straightforward data visualization tools.
In addition to selecting the right platform, it is also essential to address potential challenges in implementing big analytics in the cloud ecosystem. These challenges can include data privacy concerns, migration complexities, and talent acquisition. To overcome these challenges, organizations can implement best practices such as data encryption, phased migration strategies, and talent development programs.
Finally, it is important to foster a data-driven culture within the organization. This can include promoting data-driven decision-making, encouraging collaboration between departments, and celebrating successes in data analytics projects. By fostering a data-driven culture, organizations can ensure that they are fully leveraging the power of big analytics in the cloud ecosystem.
In conclusion, adopting big analytics in the cloud ecosystem requires careful planning and preparation. By having a clear strategy, investing in training, selecting the right platform, addressing potential challenges, and fostering a data-driven culture, organizations can successfully adopt cloud-based big analytics solutions and gain a competitive edge in their industry.
